Performance of fast learning approach to predicting black fungus diseases
Mohanad Dawood Salman
International Journal of Computational and Electronic Aspects in Engineering
Volume 3: Issue 4, December 2022, pp 70-75
Author's Information
Mohanad Dawood Salman1
Corresponding Author
1Department of Computer Sciences, College of Computer Science & math, Tikrit University, Iraq
mohanaddawoodalroomi@tu.edu.iq
Abstract:-
Microscopic examination can be used to make a preliminary diagnosis of fungal infections. Due to their apparent similarities, it frequently does not allow for the species to be identified clearly. Therefore, additional biochemical tests are typically required. This adds extra expenses and can make the identification process last up to ten days. Given the high death rate for immunosuppressed patients, such a delay in the adoption of targeted therapy could have serious consequences. The fast learning network method is an alternative that provides information with a unique approach to predicting black fungus. The experimental results of prediction showed that the performance of the fast learning method is superior as compared with the five algorithms used in this paper.Index Terms:-
black fungus, COVID-19, fast learning machine, machine learning, MicroscopicREFERENCES
- Bari, M. S., Hossain, M. J., Akhter, S., & Emran, T. B. (2021). Delta variant and black fungal invasion: A bidirectional assault might worsen the massive second/third stream of COVID-19 outbreak in South-Asia. Ethics, Medicine and Public Health, 19, 100722.
- Stone, N., Gupta, N., & Schwartz, I. (2021). Mucormycosis: time to address this deadly fungal infection. The Lancet Microbe, 2(8), e343-e344.
- Rahman, M. T., Hossain, M. G., Rahman, A. T., Huq, A. M., Farzana, S., & Nazir, K. N. H. (2021). Mucormycosis (black fungus) in COVID-19 patients—Will it be another matter of concern in the midst of the COVID-19 flare-up in Bangladesh?. Journal of Advanced Veterinary and Animal Research, 8(3), 367.
- Essar, M. Y., Khan, H., Babar, M. S., Hasan, M. M., Rackimuthu, S., dos Santos Costa, A. C., ... & Nemat, A. (2022). Mucormycosis, conflicts and COVID‐19: a deadly recipe for the fragile health system of Afghanistan. The International Journal of Health Planning and Management, 37(1), 543.
- Khan, A., Ema, N. T., Rakhi, N. N., Saha, O., & Rahaman, M. M. (2021). Parallel Outbreaks of Deadly Pathogens (SARS-CoV-2, H5N8, EVD, Black fungi) around the World in 2021: Priorities for Achieving Control with Socio-Economic and Public Health Impact.
- Vishwakarma, Y. K., Shahi, A., & Singh, R. S. (2022). Effect of Indoor Bioaerosols (Fungal) Exposure on the Health of Post-COVID-19 Patients and Possible Mitigation Strategies. COVID, 2(7), 940-951.
- Farias, L. A. B. G., Damasceno, L. S., Bandeira, S. P., Barreto, F. K. D. A., Leitão, T. D. M. J. S., & Cavalcanti, L. P. D. G. (2021). COVID-19 associated Mucormycosis (CAM): Should Brazil be on alert?. Revista da Sociedade Brasileira de Medicina Tropical, 54.
- Bhat, I., Beg, M. A., & Athar, F. (2021). A contemporary intimidation for COVID-19 patients coinfected with mucormycosis in India. J Bacteriol Mycol Open Access, 9(2), 69-71.
- Dashti-Khavidaki, S., Saidi, R., & Lu, H. (2021). Current status of glucocorticoid usage in solid organ transplantation. World Journal of Transplantation, 11(11), 443.
- Lanfranco, O. A., & Alangaden, G. J. (2016). Genitourinary tract infections. Microbiology Spectrum, 4(4), 4-4.
- Revankar, S. G., Patterson, J. E., Sutton, D. A., Pullen, R., & Rinaldi, M. G. (2002). Disseminated phaeohyphomycosis: review of an emerging mycosis. Clinical Infectious Diseases, 34(4), 467-476.
- Richardson, M., & Lass‐Flörl, C. (2008). Changing epidemiology of systemic fungal infections. Clinical microbiology and infection, 14, 5-24.
- Sen, M., Honavar, S. G., Sharma, N., & Sachdev, M. S. (2021). COVID-19 and eye: a review of ophthalmic manifestations of COVID-19. Indian Journal of Ophthalmology, 69(3), 488.
- Bhattacharyya, A., Sarma, P., Sharma, D. J., Das, K. K., Kaur, H., Prajapat, M., ... & Medhi, B. (2021). Rhino-orbital-cerebral-mucormycosis in COVID-19: A systematic review. Indian journal of pharmacology, 53(4), 317.
- Mahalaxmi, I., Jayaramayya, K., Venkatesan, D., Subramaniam, M. D., Renu, K., Vijayakumar, P., ... & Vellingiri, B. (2021). Mucormycosis: An opportunistic pathogen during COVID-19. Environmental research, 201, 111643.
- Seidel, D., Simon, M., Sprute, R., Lubnow, M., Evert, K., Speer, C., ... & Cornely, O. A. (2022). Results from a national survey on COVID‐19‐associated mucormycosis in Germany: 13 patients from six tertiary hospitals. Mycoses, 65(1), 103-109.
- Bhatt, K., Agolli, A., Patel, M. H., Garimella, R., Devi, M., Garcia, E., ... & Sanchez-Gonzalez, M. (2021). High mortality co-infections of COVID-19 patients: mucormycosis and other fungal infections. Discoveries, 9(1).
- Groll, A. H., & Walsh, T. J. (2001). Uncommon opportunistic fungi: new nosocomial threats. Clinical Microbiology and Infection, 7, 8-24.
- Chegini, Z., Didehdar, M., Khoshbayan, A., Rajaeih, S., Salehi, M., & Shariati, A. (2020). Epidemiology, clinical features, diagnosis and treatment of cerebral mucormycosis in diabetic patients: a systematic review of case reports and case series. Mycoses, 63(12), 1264-1282.
- Allamanis, M. (2019, October). The adverse effects of code duplication in machine learning models of code. In Proceedings of the 2019 ACM SIGPLAN International Symposium on New Ideas, New Paradigms, and Reflections on Programming and Software (pp. 143-153).
- Allugunti, V. R. (2022). Breast cancer detection based on thermographic images using machine learning and deep learning algorithms. International Journal of Engineering in Computer Science, 4(1), 49-56.
- L. R. Sultan, S. K. Abdulateef, and B. A. Shtayt "Prediction of student satisfaction on mobile-learning by using fast learning network," Indonesian J. Elect. Eng. Comput. Sci., vol. 27, no. 1, pp. 488-495, 2022.
- Singh, K., Kumar, S., & Kaur, P. (2019). Support vector machine classifier based detection of fungal rust disease in Pea Plant (Pisam sativam). International Journal of Information Technology, 11(3), 485-492.
- Alajas, O. J., Concepcion, R., Dadios, E., Sybingco, E., Mendigoria, C. H., & Aquino, H. (2021, June). Prediction of Grape Leaf Black Rot Damaged Surface Percentage Using Hybrid Linear Discriminant Analysis and Decision Tree. In 2021 International Conference on Intelligent Technologies (CONIT) (pp. 1-6). IEEE.
- S. K. Abdulateef, T. A. N. Abdali, M. D. S. Alroomi, and M. A. A. Altaha, "An optimise ELM by league championship algorithm based on food images," Indonesian J. Elect. Eng. Comput. Sci., vol. 20, no. 1, pp. 132-137, 2020, doi: 10.11591/ijeecs.v20.i1.pp132-137.
- Santoso, H., Tani, H., & Wang, X. (2017). Random Forest classification model of basal stem rot disease caused by Ganoderma boninense in oil palm plantations. International journal of remote sensing, 38(16), 4683-4699.
- Rahmat, R. F., Aruan, T., Purnamawati, S., Faza, S., & Lini, T. Z. (2018, September). Fungus image identification using K-Nearest Neighbor. In IOP Conference Series: Materials Science and Engineering (Vol. 420, No. 1, p. 012097). IOP Publishing.
- X. Sun, "Kinematics model identification and motion control of robot based on fast learning neural network," Journal of Ambient Intelligence and Humanized Computing, vol. 11, no. 12, pp. 6145-6154, 2020, doi: 10.1007/s12652-019-01459-z.
- Zieliński, B., Sroka-Oleksiak, A., Rymarczyk, D., Piekarczyk, A., & Brzychczy-Włoch, M. (2020). Deep learning approach to describe and classify fungi microscopic images. PloS one.
To view full paper, Download here
To View Full Paper
For authors
Author's guidelines Publication Ethics Publication Policies Artical Processing Charges Call for paper Frequently Asked Questions(FAQS) View All Volumes and IssuesPublishing with
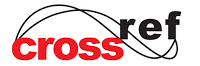
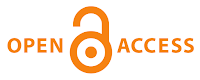
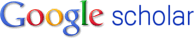
